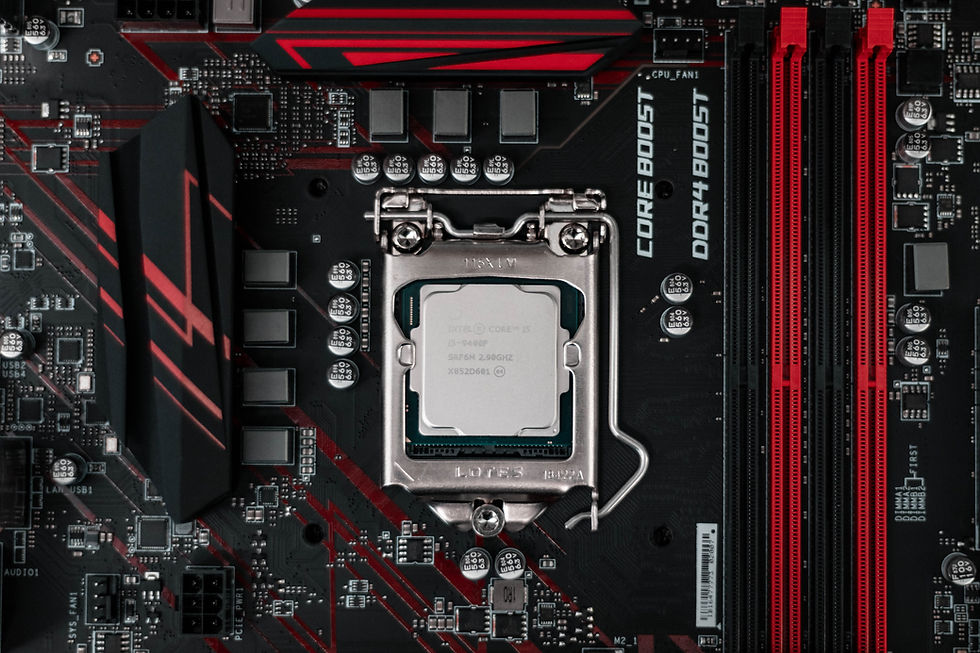
It is an industry-wide phenomenon that has opened the best opportunities for organisations across the board. Artificial Intelligence has become the growth story for India’s digital natives like Flipkart, Swiggy and Ola. Today, many AI applications — facial recognition, product recommendations, virtual assistants have become rooted in our day-to-day lives. However, these emerging AI applications have one common feature ruling them all — dependence on hardware has become the core enabler of innovation. In fact, many rising consumer digital companies are dependent on next-gen architecture that can significantly increase computational efficiency and speed the time-to-market.
According to an IDC report, spending on AI systems will more than double to $79.2 billion1 in 2022 with a compound annual growth rate (CAGR) of 38.0% over 2018-2022 forecast period. Hardware spending, dominated by servers, is projected to touch $12.72 billion this year as companies aggressively invest in building up the infrastructure necessary to support AI systems.
This has necessitated a shift towards an ‘AI technology stack’ that abstracts away the complexity of the hardware layer related to storage, memory and logic and allows higher performance gains for developers and data scientists. What we are seeing now is a new value-creation in the market by leading semiconductor companies that are focusing on end-to-end solutions for industries.
The writing on the wall is clear — the mega breakthroughs in IT aren’t going to come from hardware alone, but from the intersection of AI, hardware and software. AI hardware solutions can only deliver maximum gains if they are compatible with other layers of the software environment. To serve their customers better, semiconductor companies are developing a common programming framework and ecosystem that is in concert with hardware.
Main Features
1. No standard AI chip: The AI market is vast, but there is no “one-size-fits-all” approach. Thus, there can be no “standard” AI chip
2. Need to abstract away hardware complexity: Data Scientists and application developers look for high-performance hardware that can churn out general purpose AI solutions within a certain amount of time and power budget. They also demand increased flexibility with hardware that allows them to program with mainstream languages at a higher abstraction level along with libraries. The data science community is looking for a complete solution stack that abstracts away the hardware specifics, allowing them the ease to crunch parallel workloads more efficiently.
3. Shift to inference at scale: Inference at scale marks deep learning’s coming of age. By 2020, the ratio of training deep learning models to inference within enterprises will rapidly shift to 1:53, as compared to the 1:1 split we see today. In fact, Deloitte research predicts that by 2023, 43 percent4 of all AI inference will occur at the edge. Inference is important because it allows enterprises to monetize AI by launching new applications or products by applying their trained models to new datasets. In fact, analysts forecast that inference will be the biggest driver and is projected to generate more revenue in data centers than at the edge.
4. Rethinking data center AI infrastructure: GPUs widely known for parallel processing power are geared towards training where one inputs the data into the model. Training is also carried out in a centralized location, while inference is pushed out to the edge of the network. This requires enterprises to rethink their infrastructure strategy around training as well inference.
5. Scale-up/scale-down approach: Organizations are increasingly leaning towards a scale-up/scale-down approach where it is easy to scale up CPU clusters/processors to enable efficient power consumption without sacrificing performance, while minimizing the need for major redesign.
Intel Drives Innovation with Multi-purpose to Built AI Compute from Device to Cloud
In this article, we take a look at Intel’s AI strategy and how the chip giant has built winning roadmap for the fast-growing AI market. Further, it has created a software strategy with tools such as BigDL, nGraph and VNNI which enables developers to make maximum gains from its hardware portfolio.
Run the AI You Need on the CPU You Know, With 2nd Gen Intel® Xeon® Scalable Processors
Intel has built a new generation of hardware and software that allows enterprises to enter an era of pervasive intelligence and also address specific customer needs. Built with a data-centric focus, 2nd Gen Intel® Xeon® Scalable processors improve performance up to 277X for inference5 compared to the processor’s initial launch in July 2017.
With the growing buzz around AI/ML, 2nd Gen Intel® Xeon® Scalable processors promise an AI acceleration push, coupled with Intel® DL Boost – tailored for deep learning inferencing. With 2nd Gen Intel® Xeon® Scalable processors, Intel® DL Boost provides a winning combination without relying on GPUs. AI capabilities can be more easily integrated alongside other workloads on multi-purpose 2nd Gen Intel Xeon Scalable processors.
Furthermore, VNNI6 can be thought of as an AI inference acceleration are integrated into every 2nd Gen Intel Xeon Scalable processor. Further, performance can significantly improve for both batch inference and real-time inference, because VNNI reduces both the number and complexity of convolution operations required for AI inference, which also reduces the compute power and memory accesses these operations require.
Why is Intel® DL Boost pegged as a breakthrough?
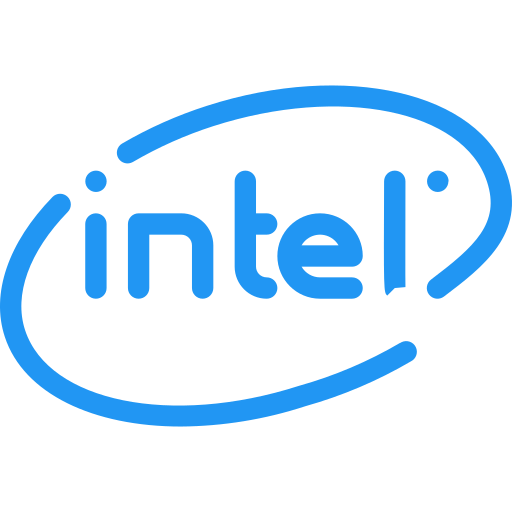
The answer is straightforward. Most commercial deep learning applications today use 32-bits of floating point precision (fp32) for training and inference workloads. However, both deep learning training and inference can be performed with lower numerical precision, using 16-bit multipliers for training and 8-bit multipliers for inference with minimal to no loss in accuracy.
With Intel® DL Boost, Intel has created a new X86 instruction that can perform an integer (INT) 8 (bit precision) matrix multiplication and summation with fewer cycles than before. Intel® DL Boost crunches 3 instructions into 17 and can speed up dense computations characteristic of convolutional neural networks (CNNs) and deep neural networks (DNNs). The main advantage for developers and data scientists is that when it comes to AI inferencing for trained neural networks that don’t require periodic retraining, one no longer needs to rely on special purpose compute hardware like GPUs or TPUs.
Here’s a benchmarking report from Dell* that emphasizes how the latest generation processor performs faster in parallel workloads, including inferencing. During benchmark testing, Dell engineers realised more than 3X8 faster inferencing for image recognition with INT8, ResNet50.
In Conclusion
What’s evident is the shift towards a general-purpose AI stack that enterprises can deploy for Deep Learning. To that end, AI computing companies need to provide a full-stack solution across silicon, tools and libraries for easier application development. Meanwhile, the developer ecosystem demands SDKs and compilers to optimise and accelerate AI algorithms.
There’s a need to bring more AI capabilities to enterprises and empower developer ecosystem. We believe Intel is playing a pivotal role in the emerging AI market with its end-to-end solutions. Intel has a wide array of AI hardware that includes CPUs, accelerators/ purpose-built hardware, FPGAs and in the future neuromorphic chips. Developers look for a software environment that can function across different platforms without them having to overhaul their systems.
On the software side, Intel is winning the market with tools like Intel® Distribution of OpenVINO™ Toolkit – that accelerate deep neural network workloads and optimizes deep learning solutions across various hardware platforms. In addition to this, support for popular Deep Learning frameworks like TensorFlow* and MXNet*, machine learning libraries like PyTorch* and existing compilers like ONNX* will help the chip giant win developer mindshare.
Comments