Data Mining:
Data mining is a process of extracting the hidden predictive information from the extensive database.
Data mining is used by the organization to turn raw data into useful information.
The term "data mining" is a, misnomer, because the goal is the extraction of patterns and knowledge from large amounts of data, not the extraction (mining) of data itself. It also is a buzzword and is frequently applied to any form of large-scale data or information processing (collection, extraction, warehousing, analysis, and statistics) as well as any application of computer decision support system, including artificial intelligence (e.g., machine learning) and business intelligence.
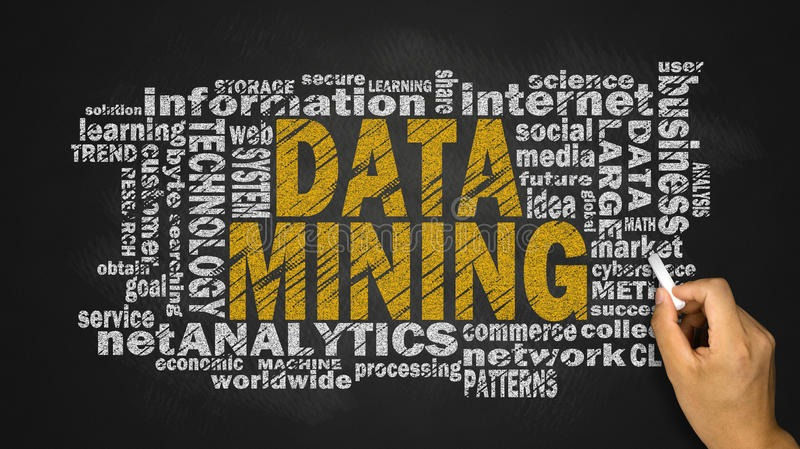
The actual data mining task is the semi-automatic or automatic analysis of large quantities of data to extract previously unknown, interesting patterns such as groups of data records (cluster analysis), unusual records (anomaly detection), and dependencies (association rule mining, sequential pattern mining)
This usually involves using database techniques such as spatial indices.
Data mining involves six common classes of tasks:
Classification – is the task of generalizing known structure to apply to new data. For example, an e-mail program might attempt to classify an e-mail as "legitimate" or as "spam".
Regression – attempts to find a function that models the data with the least error that is, for estimating the relationships among data or datasets.
Summarization – providing a more compact representation of the data set, including visualization and report generation.
Anomaly detection – The identification of unusual data records, that might be interesting or data errors that require further investigation.
Association rule learning – Searches for relationships between variables. For example, a supermarket might gather data on customer purchasing habits. Using association rule learning, the supermarket can determine which products are frequently bought together and use this information for marketing purposes. This is sometimes referred to as market basket analysis.
Clustering – is the task of discovering groups and structures in the data that are in some way or another "similar", without using known structures in the data.
Proprietary data-mining software and applications:
The following applications are available under proprietary licenses.
NetOwl: suite of multilingual text and entity analytics products that enable data mining.
Oracle Data Mining: data mining software by Oracle Corporation.
PSeven: platform for automation of engineering simulation and analysis, multidisciplinary optimization and data mining provided by DATADVANCE.
Qlucore Omics Explorer: data mining software.
RapidMiner: An environment for machine learning and data mining experiments.
SAS Enterprise Miner: data mining software provided by the SAS Institute.
SPSS Modeler: data mining software provided by IBM.
STATISTICA Data Miner: data mining · software provided by StatSoft.
Tanagra: Visualisation-oriented data mining software, also for teaching.
Angoss KnowledgeSTUDIO: data mining tool
LIONsolver: an integrated software application for data mining, business intelligence, and modeling that implements the Learning and Intelligent OptimizatioN (LION) approach.
Megaputer Intelligence: data and text mining software is called PolyAnalyst.
Microsoft Analysis Services: data mining software provided by Microsoft.
Goal of data mining:
Prediction - Data mining field focus on prediction compare to generate exact results for future purpose.
Identification - identification allows us to identify the data pattern in the existing item.
Classification – Data mining can partition the data into classes for example supermarket.
Optimization – The one goal of data mining can optimize the use of limited resources like time, space, money, or material.
Comentarios